Five years ago, we launched the first high-stakes, digital-first test that could be taken anytime and anywhere in the world, because we saw how technology could radically improve testing for students and institutions. In this five part series, we take a look back at the enormous amount of research and development that went into reinventing the world of high-stakes testing.
A fair shake
We all agree that tests should be fair. But what does that actually mean? Simply put, a fair test gives all test takers an equal chance to demonstrate the skill, ability, or proficiency in what assessment scientists call the “construct” that the test is intended to measure.
On any language test, factors like age, gender, or nationality have the potential to affect how individuals perform, due to varying degrees of familiarity with cultural norms, subject matter, and vocabulary that make up the test’s “items”—that is, the questions, tasks, and prompts that test takers respond to.
If people with the same proficiency level don’t have equal likelihood of performing well on a test, it may suffer from measurement bias: something about the exam may give some test takers an unfair advantage.
For example, Indian test takers, on average, are more familiar with cricket than test takers from a number of European countries. So Indian test takers may be more likely to say more when describing a picture or responding to a prompt about cricket—even after accounting for differences in English proficiency.
This isn’t to say that factors like cultural familiarity always lead to measurement bias, but they sometimes can. Because the Duolingo English Test is taken by people all over the world (in 207 countries and territories and counting!) it's important to ensure that differences in things like cultural background or first language don’t interfere with people’s chances of success.
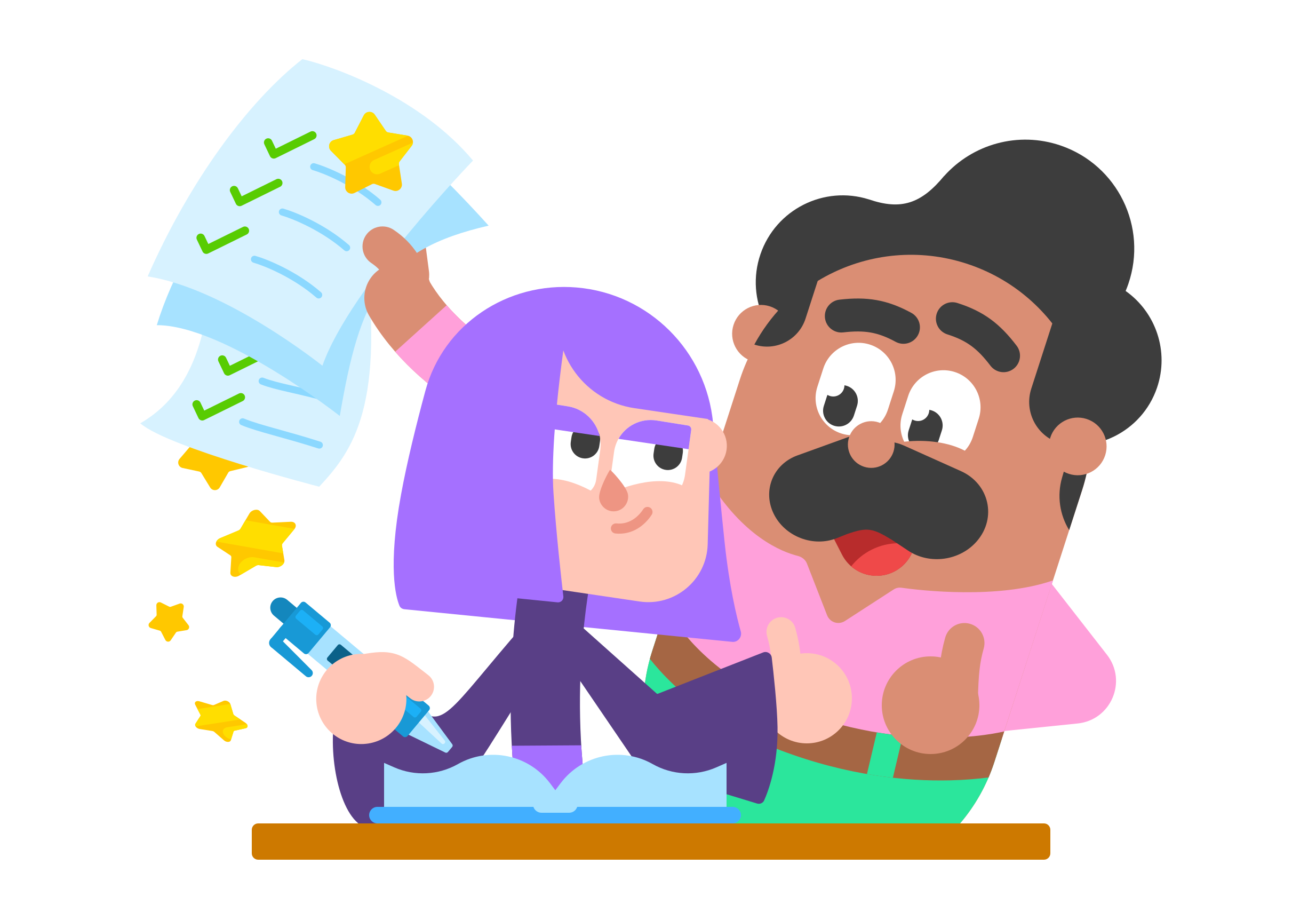
What's the DIF
Before an item is added to the Duolingo English Test item bank, it undergoes a rigorous review process during which our assessment scientists evaluate it for fairness and bias. But humans aren’t perfect; no matter how objective we aim to be, we each have our own biases, too—even experts!
To confirm that the items we believe to be fair aren’t being affected by factors beyond language proficiency, our assessment scientists also analyze the items after the test is administered for something called “Differential Item Functioning,” or DIF: evidence of different groups of people having a different propensity to respond to an item correctly, even when their English proficiency (as indicated by their overall score) is the same.
For decades, the testing industry’s approach to DIF has been to look at individual items to see whether, for test takers with the same score, the distribution of responses may be affected by factors such as age, nationality, and native language. These groups are analyzed one at a time, independently of the other variables being examined.
But we know that this isn’t how the world works—people vary in an infinite number of dimensions beyond English proficiency (like first language and interest in cricket) and belong to multiple demographic categories simultaneously. So a single-variable approach to DIF analysis, while better than nothing, doesn't capture the big picture, and might let some instances of measurement bias go unnoticed.
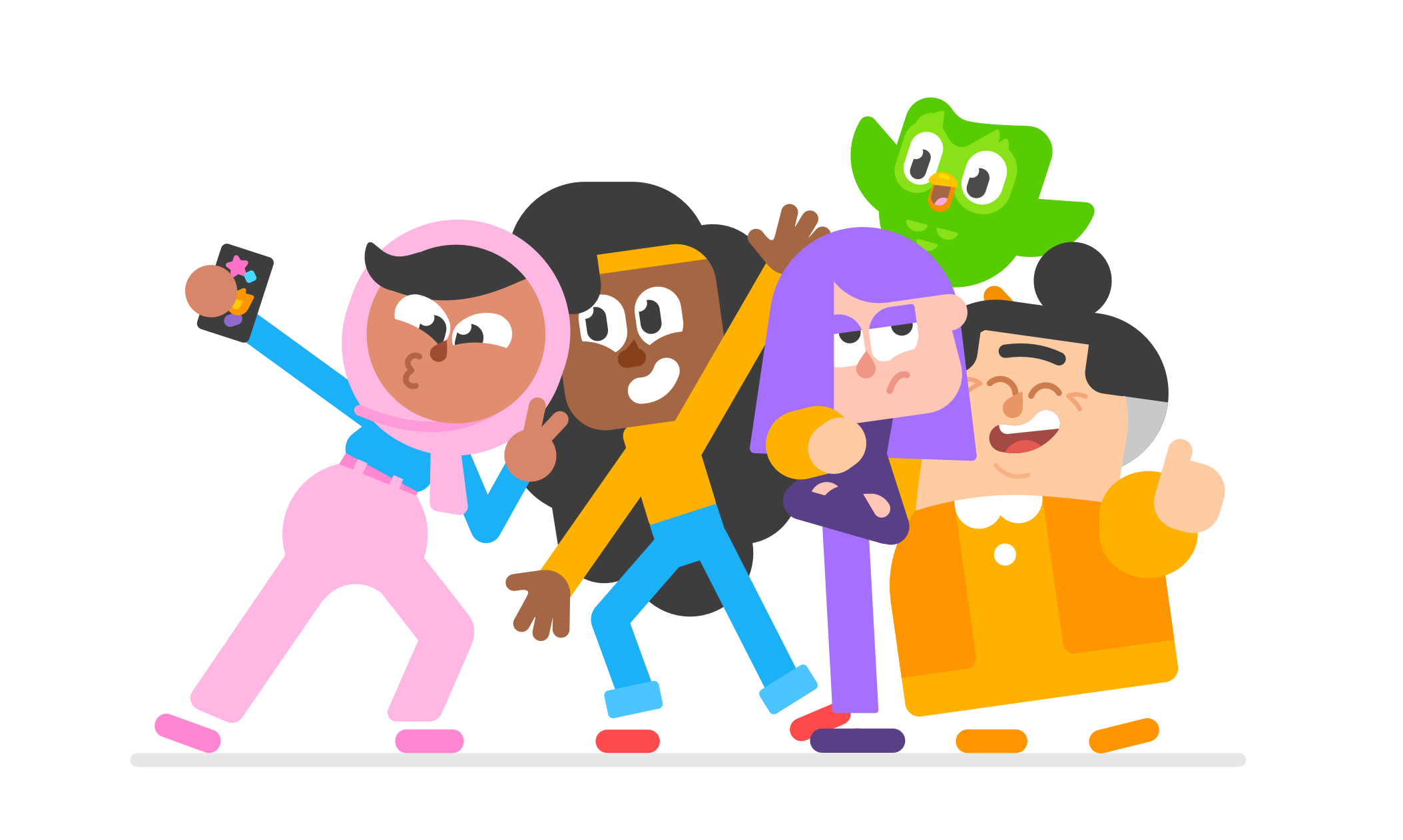
More than the sum of their parts
At Duolingo, we recognize that test takers are more than a collection of demographic variables. That’s why we use a multidimensional, person-centric approach to DIF analysis.
For example, age and gender can contribute to measurement bias separately, and different combinations of ages and genders can contribute to bias; because of this, certain items might result in more DIF for certain combinations of age and gender. So in our DIF analyses, we look at how age affects item responses for test takers of the same gender, and we also analyze how responses vary for different genders in each age group.
“It’s a far more sophisticated way to analyze fairness,” explains Dr. Will Belzak, a psychometrician on our Assessment Research team who pioneered this integrative new approach. “We’re not just looking at a single dimension in isolation of itself, we’re looking at how multiple dimensions can interact in complex ways to bias a test question.”
Because the Duolingo English Test can be taken anywhere in the world on any computer with an internet connection, we also analyze DIF beyond the traditional demographic categories, for example, checking image-based items for variance across screen size to ensure that item responses aren’t affected by the devices people are using to take the test.
If we detect that an item response rate varies across different groups of test takers, that item is flagged for DIF and retired from the test’s item bank, so that a panel of content experts can further analyze it.
Doing testing better
Ultimately, because humans create tests, there’s no way to escape bias entirely. However, by leveraging AI and statistical methods, our experts in assessment development can be more systematic in detecting unfairness, and more strategic when correcting for it.
“Our method is much more sensitive to smaller effects of bias than what’s been done in the past,” says Belzak. “The modern psychometric approach we are taking in our DIF analysis is just one of the ways we are able to do testing better.”
To learn more about our approach to DIF analysis, check out this paper co-written by Dr. Will Belzak, published in Psychological Methods.