When I began writing on ethics and AI in the late 2010s, most of the articles I read focused on Algorithmic Bias, noting that the data used to train algorithms did not include a full and balanced representation of different segments of the population.
For example, when Joy Buolamwini and Timnit Gebru investigated the racial, skin type, and gender disparities embedded in commercially available facial recognition technologies, they revealed how those systems largely failed to differentiate and classify darker female faces while successfully differentiating and classifying white male faces. The poor classification for darker female faces stemmed from the data sets used to develop the algorithms, which included a disproportionately large number of white males and few Black females. When they used a more balanced data set to develop the algorithm, it produced more accurate results across races and genders.
I agree that algorithmic bias is important, but it is not the whole story.
The work of my collaborators and me on ethical AI in Education (AIED) stands out for its holistic approach. In our recent publication, "The Cyclical Ethical Effects of Using Artificial Intelligence in Education," Chris Dede, Beth Holland, Michael Walker, and I conduct a synthetic review on the ethics and effects of using artificial intelligence in education, which reveals Five Key Factors in AI's Ethical Landscape associated with access, representation, algorithms, agency and interpretations, and citizenship depicted in Figure 1.
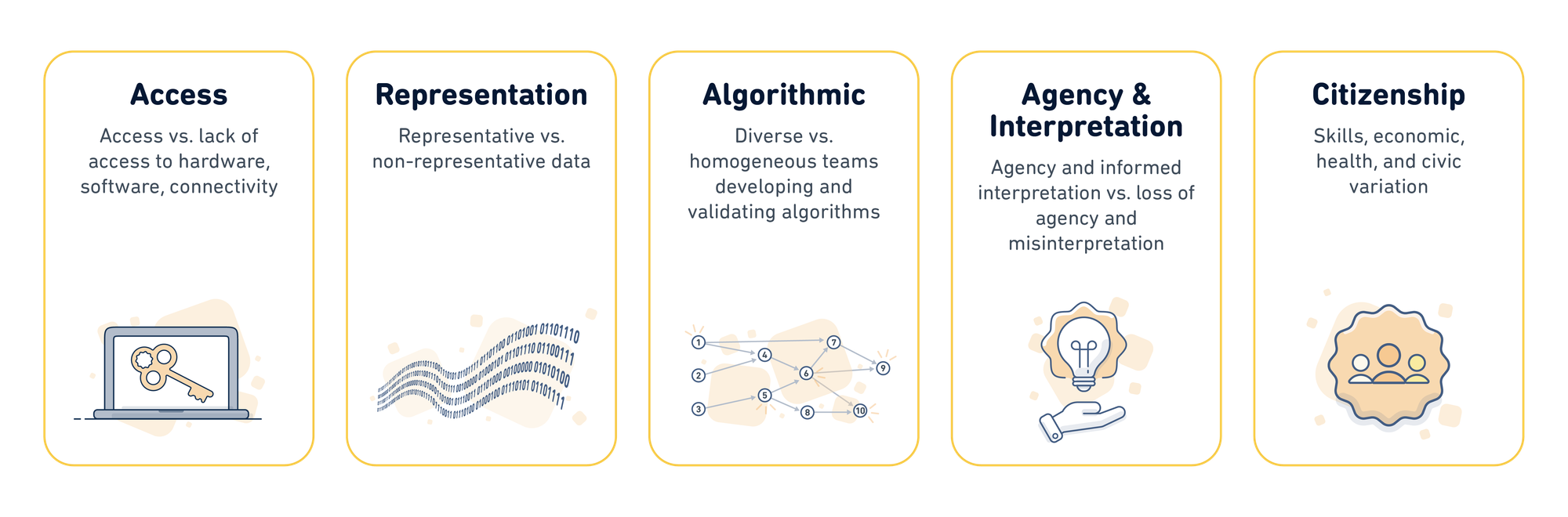
Virtuous and Vicious Cycles
These factors can create divides and ultimately cycles in which some categories of people benefit unduly, and others lose out, as depicted in Figure 2. For those who lose out due to discrimination, unequal access to power and opportunity, and other unfair or unjust practices, the divides can create a vicious cycle that perpetuates and potentially amplifies structural biases in teaching and learning, with significant impacts on life outcomes, such as education and employment, health and well-being, relationships and social connection, and personal development and fulfillment.
However, increasing human responsibility and control over these divides can create a virtuous cycle that improves diversity, equity, and inclusion in education and increases the likelihood of positive impacts on the same life outcomes.
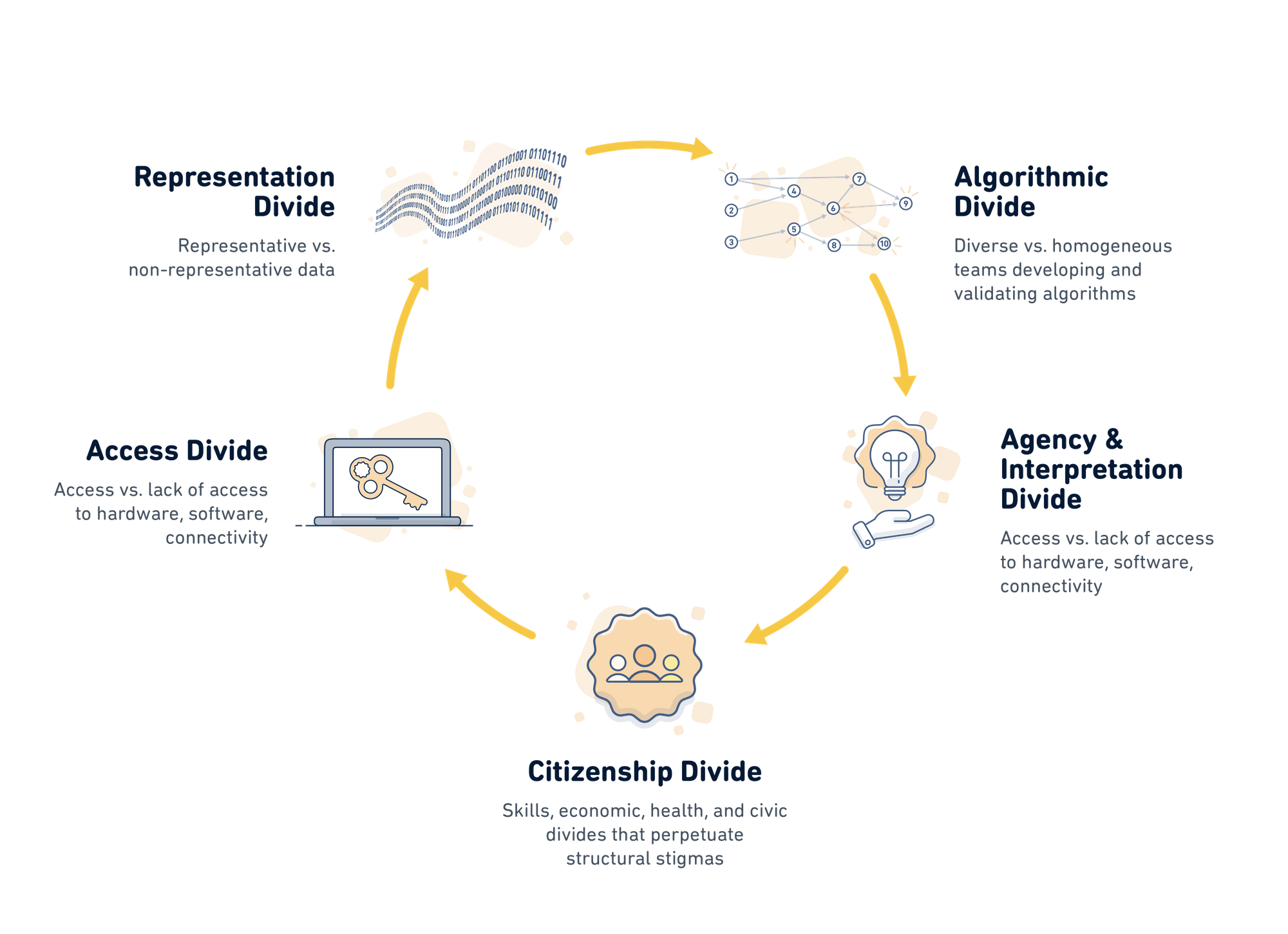
Imagine a new technology that claims it supports Personalized Learning and increases student success by centering on the learner and recognizing that learning extends beyond the classroom and flourishes through meaningful connections with peers, mentors, and the community. Sounds great, right? But before we embrace this or any AI-enhanced solution, there are important questions we as educators, researchers, developers, and policymakers need to ask. In this light, Duolingo's approach to AI in education presents an intriguing case study.
Duolingo, a prominent player in the intersection of AI and education, acknowledges the powerful synergy between the two realms. Their commitment to making world-class education accessible aligns with our own pursuit of a holistic approach to ethical AI in education (AIED). Their recent article, 3 Ways Duolingo Improves Education Using AI, describes how they use AI to create personalized and engaging educational experiences for millions worldwide.
Critical Questions Before Embracing AI in Education
First, we must interrogate the creators of AI solutions. Who built the AI engine? Were diverse voices, perspectives, and disciplines present in its design and development? If not, we risk replicating the very biases identified by Buolamwini and Gebru.
Beyond the creators, the data itself demands scrutiny. Two areas pose immediate challenges:
- Access Disparities in Tech Tools and Internet Connectivity: Not everyone has the same tech tools or internet connection, which can put some students at a disadvantage. Who does and does not have access to the hardware, software, and connectivity necessary to engage with AI-enhanced digital learning tools and platforms? If students do not have access, they fail to contribute to the datasets that are used to build AI systems.
- The Role of Accurate and Representative Data in AI Development: If the information used to build AI tools is inaccurate or isn't fully representative of the population who will use the tool, it can lead to unfair decisions and missteps. What factors make data either representative of the total population or over-representation of a group's preferences, thereby preventing objectivity and biasing understandings and outcomes?
But it's not just about the tech itself. It's also about how we understand and use the information that comes from the AI system. We must carefully consider how we interact with the information AI generates once the technology exits the lab and heads into the classroom.
Moving Beyond Tech: Monitoring the Cumulative Effects
Blindly trusting AI predictions without human analysis can limit student opportunities. How can educators, students, and administrators understand and utilize AI outputs (dashboards, reports, recommendations) wisely for informed decision-making?
The cumulative effect of these divides shapes student behaviors, skills, culture, and even future life paths. How can we continuously monitor and address access, data bias, and interpretation to ensure ethical, equitable AI use in education? In our exploration, we underscored the role of human agency in interpreting AI outputs wisely.
Holistic Ethics in AI Education: Beyond Algorithmic Bias
AI in education requires a holistic approach to ethics. Simply addressing algorithmic bias is insufficient. We must consider access, representation, human agency, interpretable algorithms, and democratic citizenship. These elements intertwine, potentially creating vicious cycles of inequity. Duolingo's proactive stance in developing and sharing Responsible AI standards for their English Test sets a commendable precedent in the digital-first assessment landscape.
Breaking these cycles demands constant critical reflection and iterative improvement. Educators and AI designers must continually assess who benefits and who loses at each stage. Proactive steps are essential to fostering inclusion and equity. This includes expanding access, improving data representation, building interpretability into algorithms, empowering human agency, and shaping technology for democratic citizenship.
Fostering Inclusion and Equity in AI Education
Humility is key to recognizing our own biases and the unintentional obstacles we introduce. No individual or organization holds all the answers. Progress hinges on cross-disciplinary collaboration and amplifying marginalized voices. Together, we can harness the power of AI to create a more just, equitable, and empowering learning experience for every student. But this future depends on maintaining a holistic perspective on AI ethics, engaging in continuous critical reflection, and always prioritizing equity and human agency.
Duolingo's commitment to responsible AI standards for the DET resonates strongly with our call for a holistic ethics approach in AI education. They recognize the evolving nature of generative AI and advocate for clear guidelines encompassing accountability, transparency, fairness, privacy, and security.
Look out for my upcoming blog, where I'll explore why researchers are uniquely poised to serve as ambassadors of AIED.
Read the complete article, The cyclical ethical effects of using artificial intelligence in education in AI & Society.